The State of ARD for Geospatial Missions
With trillions of pixels of data captured every day by satellites, there is a vast amount of information available. However, not all satellite data is as accessible or suitable as one may think. Geospatial data often requires significant pre-processing and preparation before it can be used for analysis, demanding a high level of technical expertise. The pursuit of analysis-ready data (ARD), aims to overcome these challenges by making datasets more readily available and accessible for a broader range of users, opening up new opportunities for geospatial data applications.
What is ARD?
Analysis-ready data (ARD) refers to data that has already been “cleaned” or prepared for use. The goal of ARD is to make accessible data that is coherent, easily understood and ready for analysis with minimal additional processing, while enabling interoperability between datasets. One aspect of this is ensuring that the data is machine readable in a standard format. Earlier methods consisted of sharing raw data as a pdf file in pure text format. This took extensive amounts of time to translate to a usable form. Similarly, ARD is data that is already computed in a state that is validated in a standard measurement context. This means that the data has been determined as suitable for accurate measurement.
Another aspect of ARD is data summarization. Metadata such as footprints and aggregate value statistics can help users know whether or not they actually need to analyse a particular data set, saving time and resources. Additionally, ARD is annotated with quality assessment flags that indicate where errors may arise, such as clouds or shadows. This enables analysts to quickly scan for discrepancies, ultimately enabling more efficient analysis.
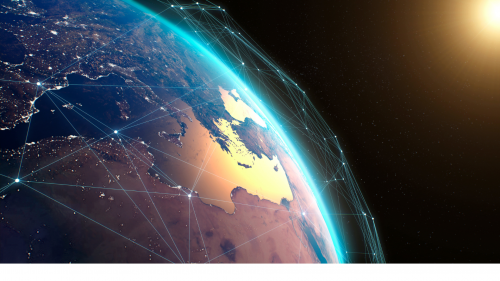
ARD in the Geospatial Industry
Within the geospatial industry, ARD enables seamless integration and analysis of satellite data sets through a standardized format and coordinate system. Ultimately, ARD would make commercial satellite data easier to access and use, especially for non-specialist users. With ARD, satellite datasets would include consistent file formats, projections, and coordinate systems across sensors. Consistent, coherent, and compatible data enhances users ability to effectively monitor changes over time with data from multiple satellite sensors or time periods. ARD will also save time and resources that would otherwise be directed towards data processing and preparation such as geometric corrections, enabling analysts to focus their time on doing better analysis. Ultimately, this compatibility will encourage users to choose standardized ARD datasets.
There have been a number of implementations pursuing the concept of ARD. Despite this, the problem that arose was one of isolation, with each mission working independently. This resulted in fragmentation across the geospatial industry between each specification.
Existing ARD Efforts
The United States Geological Survey (USGS) was the first to develop ARD products for public missions with Landsat and Sentinel. As the first, they were able to design the foundational standards for ARD. Originally intended for internal use, the ARD product was designed to work for their individual processes. As the datasets became more openly available, USGS transitioned to a cloud-native approach, storing their satellite data in specific file types.
From the commercial side of the industry, Maxar and Planet followed suit, keeping to their own proprietary standard and customizing for their own datasets. This included addressing metadata and masking requirements.
Committee on Earth Observation Satellites (CEOS), has made the most concerted effort to standardize ARD, by focusing primarily on use cases within academia. Satellite data is processed to a minimum set of requirements and organized in a way that enables immediate analysis with minimal user effort. Additionally, there is a level of interoperability through time and space with other datasets. This framework has provided new possibilities for operator datasets to be utilized.
Limitations
Despite efforts to pursue ARD, there are some limitations to current standards. To begin, reliability of the data depends on up to date, precise calibration. Without periodic validation that the calibration is still “correct”, accuracy details included in ARD data products lose their reliability. There are many different ways that each individual operator chooses to calibrate and validate their data, which can lead to inconsistencies when utilizing multiple data sources. Additionally, there are high accuracy requirements with limits on the type of reporting, which cause issues for some sensors. For example, CEOS ARD requires all methods and tools to be disclosed. This can conflict with confidential processes among some suppliers, resulting in hesitance when adopting that specification.
In current ARD processes there is also a low consideration for how the data will actually be utilized. For example, the requirement to be “machine readable” does not specify how. Data sets may be compliant, but two different methods could be used, defeating the standardization aspect of ARD. Consistent schemas and formats are critical. ARD being different between sources only exacerbates the issue by taking the problem we had and simply putting a bandage over it.
What Arlua is Doing
The Arlula team has recently embarked on a project to address these limitations. A key goal of our efforts is to enforce the usage of cloud-optimized formats and well defined schemas, particularly around STAC. This has extension based modality, which makes it easier to extend the standard without having to rewrite from scratch. Additionally, we acknowledge that unique use cases require different standards and specifications, and we aim to create a specification that prioritizes utility. This would include a set of minimal actionable requirements that operators can comply with while maintaining confidentiality. And finally, we are exploring solutions for redundant metadata storage such as capture date, to help mitigate common problems faced by new users.
The push for analysis-ready data marks a shift in how geospatial data is managed and utilized, offering a significant promise for adoption in a broader range of applications and efficiency in analysis. While ARD initiatives have made significant impact by improving standardization and interoperability, challenges still remain. At Arlula, we are on a mission to take steps forward in ARD development, to unlock a range of new applications and potential for geospatial data.
Want to keep up-to-date?
Follow us on social media or sign up to our newsletter to keep up to date with new product releases and case studies.